Manual Review is the process of human reviewers painstakingly reviewing documents collected as part...
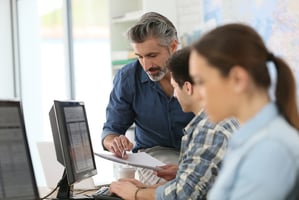
Altlaw works with various legal entities, law firms, government legal departments and company in-house counsel. Due to this, we regularly handle highly sensitive case content.
While time and costs are usually at the forefront of our concerns with eDiscovery matters, ensuring documents aren’t accidentally produced — or omitted — is of even greater importance when highly sensitive case content is involved.
With this in mind, a thorough QC process is essential. Here’s how we applied AI tools to ensure a rigorous QC process for a large DSAR case.
Our client wanted us to ensure a thorough QC process so miscoded or omitted documents with potential relevance aren't missed.
DSAR cases are particularly communication-heavy, making them ideal candidates for the sentiment analysis tool. Throughout this DSAR case, we followed a similar methodology to that described in a previous case study.
In this case, the sentiment analysis tool was appropriate to test
the documents discarded throughout the review process to ensure maximum quality control.
Documents tagged as not relevant were held in a discard pile that was later subjected to concept clustering. Once we had distinct clusters of documents that appeared to be irrelevant to the case, we overlaid the clustering with sentiment analysis.
Sentiment analysis uses natural language processing (NLP), machine learning (ML) and artificial intelligence (AI) to analyse and determine the sentiment or emotion expressed in text or speech.
The tool can configure whether the overall sentiment is positive, negative or neutral. Or, in the case of RelativityOne software, either of the following sentiments: positivity, negativity, anger and desire.
In this case, we searched for all sentiments but paid particular attention to those flagging for anger or negativity.
Using these two tools in conjunction with one another allowed us to view clusters at a glance and determine whether they contained any emotions we highlighted as important. Given the nature of DSARs, the emotions we typically scan for are anger and negativity.
However, depending on the subject matter, this isn’t always the case. Within clusters where we found evidence of anger and negativity, we could pull out documents and review them for relevance before making any necessary adjustments to coding decisions.
This method allowed us to quickly and efficiently isolate potentially relevant documents that had either been miscoded, skipped over or discarded after a cluster had initially been deemed irrelevant. By doing this, more than 20 relevant documents were prevented from being omitted.
It’s a great example of how we use initiative and industry knowledge to combine two AI tools to produce the most accurate results.
Stay up to date with all things eDiscovery and information governance. Weekly updates ensure you’re always in the loop.
Manual Review is the process of human reviewers painstakingly reviewing documents collected as part...
Ever since Relativity announced their new GenAI aiR suite of tools based on OpenAI's GPT-4 model,...
Since the launch of Chat GPT in November 2022, there has been an unprecedented uptake in the use of...